Setting the Record Straight on Predictive Policing and Race
In a thoughtful and poignant piece in the New York Times, Bärí A. Williams described her concerns about racial bias in predictive policing software and the effect such software might have on her own family. In response, Andrew Guthrie Ferguson published an excellent article on In Justice Today that clarified some of the points raised in Ms. Williams’s […]
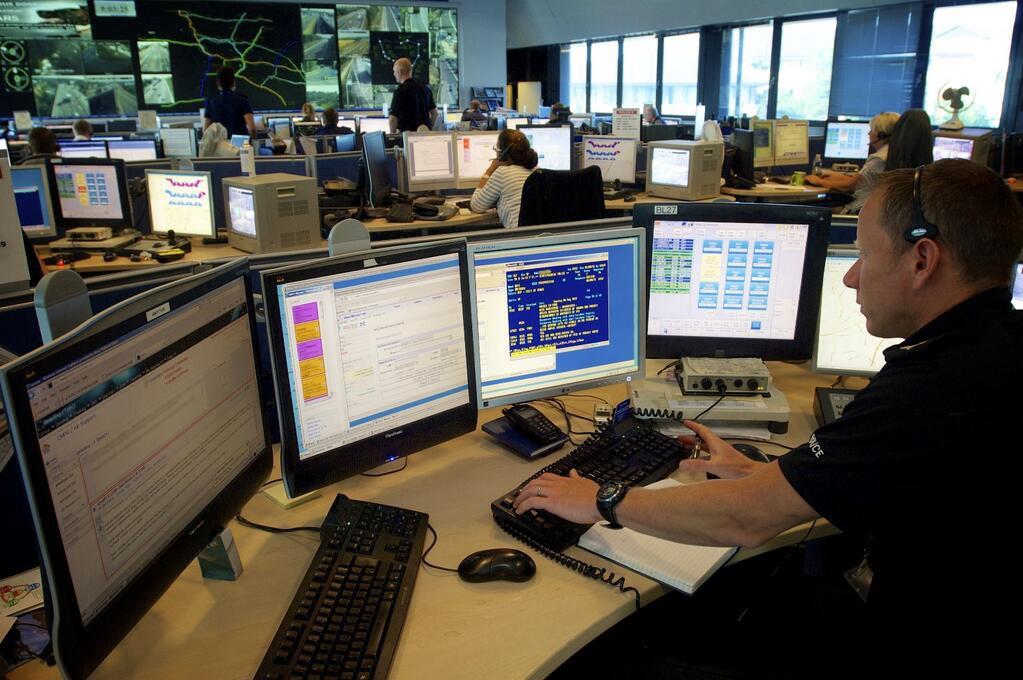
In a thoughtful and poignant piece in the New York Times, Bärí A. Williams described her concerns about racial bias in predictive policing software and the effect such software might have on her own family. In response, Andrew Guthrie Ferguson published an excellent article on In Justice Today that clarified some of the points raised in Ms. Williams’s article, including a discussion of our study about the potential impact of predictive policing in Oakland, California. Our study demonstrated the potential for predictive policing software to perpetuate historical biases in enforcement. Professor Ferguson describes our study as “hypothetical” because the algorithm we used isn’t typically used for drug crimes and Oakland doesn’t use the software. While Professor Ferguson raises some interesting points, we would like to take this opportunity to provide more context to our decision to focus on drug crimes in Oakland in our study and further unpack the broader conclusions of our study.
Critics of our study have previously raised questions about the appropriateness of using drug crime data for generating forecasts using Predpol’s software, as Predpol has not been used for forecasting drug crimes. However, this claim misses the critical argument of our study and ignores recent attempts by both police departments and the federal government to further expand the scope of place-based predictive policing to include drug crimes.
The key point of our Predpol study is that virtually all predictive policing models (even person-based models such as Chicago Police Department’s heat list) use crime data from police departments. But police department data is not representative of all crimes committed; police aren’t notified about all crimes that happen, and they don’t document all crimes they respond to. Police-recorded crime data is a combination of policing strategy, police-community relations, and criminality. If a police department has a history of over-policing some communities (which often tend to be communities of color) over others, predictive policing will merely reproduce these patterns in subsequent predictions. This is true even for models that claim racial neutrality because they say they do not explicitly include race variables in their model (which Predpol does on their website).
To demonstrate this point in our study, we needed to illustrate how predictions generated by a predictive policing algorithm (in this case, Predpol’s algorithm) compared against an alternative, likely more accurate, representation of the locations of all crimes committed, including those which are not observed by police. As we point out in our study, we chose drug crimes because it allowed for the use of public health data on illicit drug use to serve as a point of comparison. For other categories of crime, such as property crimes or violent crimes, it is more difficult to establish alternative points of comparison.
Unfortunately, critics’ focus on the type of data used in the analysis distracts from the broader point that the collection of police recorded crime data, regardless of specific crime category, is inherently biased by the institutional context under which it is collected; those biases will be perpetuated, in turn, by predictive policing models that rely on police-recorded data. Further, although PredPol may not be used to police drug crimes, it certainly has been used by some jurisdictions to allocate resources to police other crime types that suffer from similar statistical bias induced by the highly discretionary nature of enforcement. For example, in Weapons of Math Destruction, author Cathy O’Neil details the UK city of Kent’s use of the PredPol software to predict nuisance crimes and found that, much like in our study, the software suggested the same set of locations over and over again.
Lastly, despite the larger predictive policing vendors such as Predpol claiming not to predict the location of drug crimes, there is an active push by governments to do so. For example, a recent National Institute of Justice crime forecasting challenge offered over $1.2 million in cash prizes for teams that could best predict the locations of future categories of crimes. Among these groups to be predicted was “street crime,” one subcategory of which is vice crime, which includes drugs, gambling, prostitution, etc. In fact, teamsled by Predpol co-founder George Mohler (team PASDA) and Hunchlab Lead Data Scientist Jeremy Hefner won multiple cash prizes in the competition using their models to predict the location of these crimes. Even if PredPol and similar software is not currently being deployed to predict drug crimes, it is clear that people closely associated with the organizations are not on principle opposed to doing so — given their participation in developing software for the NIJ challenge to do exactly that. Cities such as Hamden, CThave explicitly requested that Predpol and other commercial predictive policing vendors submit bids for a contract to predict gang activity and drug crimes. And, with the increased concern within the Trump administration about the growing opioid crisis, it is reasonable to assume that more cities will look to predictive policing to grapple with this issue.
As to Professor Ferguson’s point about Oakland’s use of Predpol, it ignores the reality that in their Fiscal Year 2015–2017 budget, the city of Oakland had, in fact, approved $158,400 over two years for the Oakland Police department to purchase and implement Predpol. However, a report from Motherboard foundthat the police department canceled the contract after an internal committee could not find credible evidence that Predpol reduces crime, combined with the committee’s concerns — substantiated by studies such as ours — that predictive policing could have a disproportionate impact on minority neighborhoods.
This raises a critical point often missed in the debate about either person or place-based predictive policing: Even if we hypothetically had bias-free data, would it reduce crime? In their highly regarded report on predictive policing, the technology think tank Upturn points out that, “although system vendors often cite internally performed validation studies to demonstrate the value of their solutions, our research surfaced few rigorous analyses of predictive policing systems’ claims of efficacy, accuracy, or crime reduction.” The only study to finding meaningful crime reduction is the study conducted by Predpol itself in Los Angeles and Kent (UK). But, as the Motherboard articlepoints out, the reduction in crime claimed in the study may have been spurious, as LAPD’s crime statistics show other divisions that were not using Predpol also saw crime reduction as high as 16 percent during the same period.
We appreciated Professor Ferguson’s nuanced take on the different risks of each type of predictive policing software, and we agree that Chicago’s heat list is very concerning from a human rights perspective. However, it is naive to believe that predictive policing vendors will be able to self-regulate merely by voicing concerns about the potential harms of these tools. What is needed are clear guidelines for algorithmic transparency and accountability, which allow independent groups to evaluate the efficacy and potential harms of predictive policing and other algorithmic tools. Currently, many vendors view their algorithms as proprietary technology and are allowed by police departments to have opaque rule and procedures that govern which researchers or groups are permitted to conduct evaluations of their technology.
But the status quo is beginning to change. It was only because researchers associated with PredPol published an academic paper that included their algorithm — a move towards transparency we applaud — that we were able to complete our study. Further, many agencies have sought to move away from third-party commercial vendors and opt for tools built in-house or in collaboration with universities that make their source code and evaluations public. Newer predictive policing companies such as Civicscape have committed to algorithmic transparency by publishing a version of their source code on the online code repository Github, and pledged not to use their tools to predict drug crimes because of concerns that the bias present in crime data is too difficult to model out of their predictions. At the legislative level, New York City recently passed a bill to create a task force to evaluate the city’s use of automated decision systems, with the aim of eventually creating procedures by which agencies could provide source code and testing for all systems such as predictive policing. Hopefully, efforts like these will become the norm and will help communities across the country feel more confident in the potential of using data and machine learning to address pressing public safety issues.