Risk Assessment: Explained
Over the past decade, a growing number of cities, counties and states have recognized the profound injustice of a cash bail system, in which people who can afford bail walk free while those who can’t are detained. But that awareness gives rise to a thorny question: What should replace it? How should judges decide whom […]
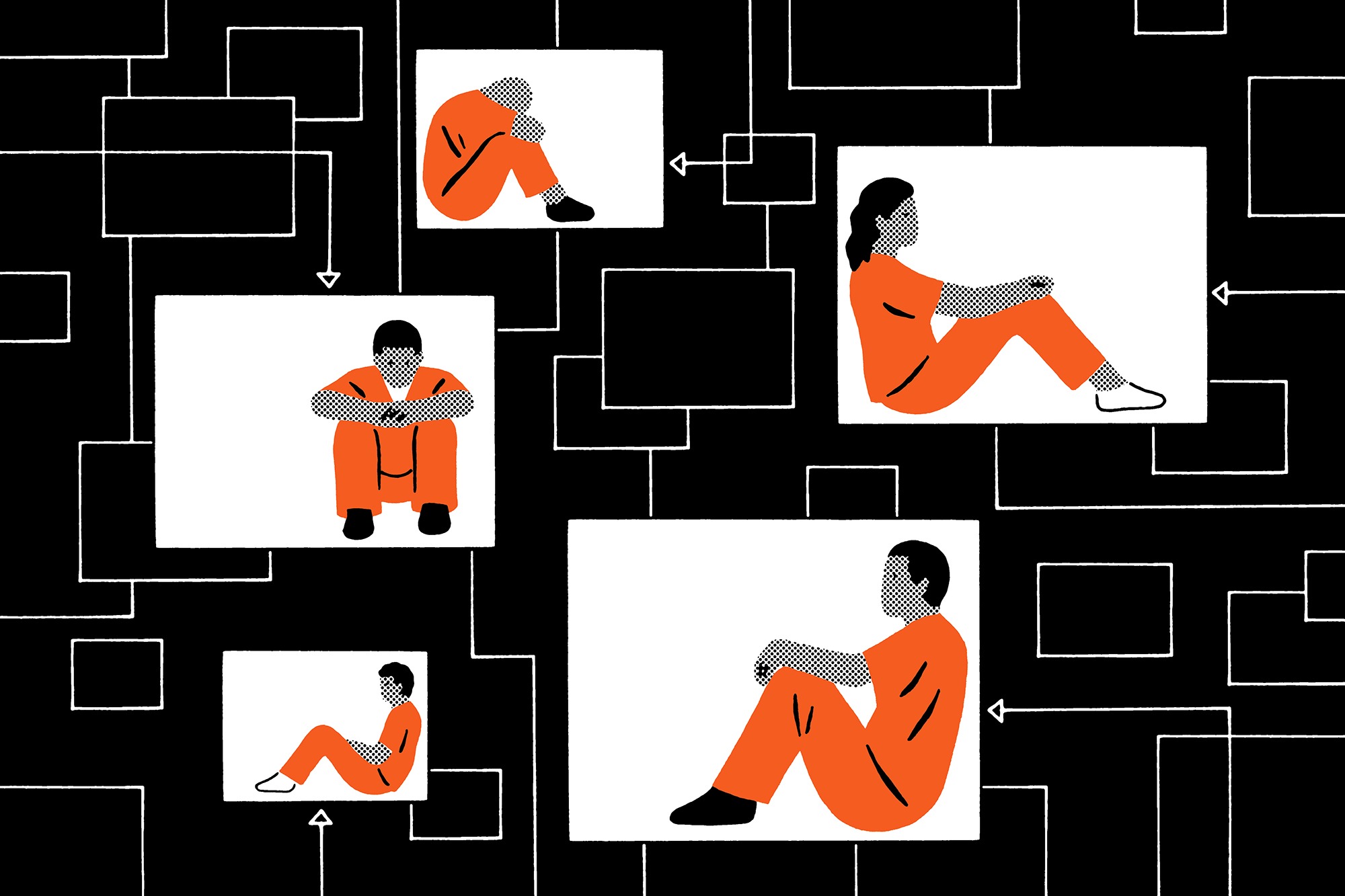
Over the past decade, a growing number of cities, counties and states have recognized the profound injustice of a cash bail system, in which people who can afford bail walk free while those who can’t are detained. But that awareness gives rise to a thorny question: What should replace it? How should judges decide whom to detain pretrial? In many cases, the answer has been to rely more heavily on risk assessments, algorithmic tools based on predictive analytics.
Not only are risk assessments used by judges in pretrial decisions, but they’re also being used or considered for use in sentencing and parole decisions. Nearly every U.S. state and the federal system have implemented risk assessment in some form. Several states are at various stages of rolling out new tools and the recently enacted First Step Act mandates the development of a new federal risk assessment meant to reduce recidivism and connect incarcerated people with services. Any corner of criminal law yet unchanged by predictive models will most likely not remain so for much longer.
It is easy to see the appeal of risk assessments. The decisions that judges and other justice system actors make daily can be hugely consequential, not just for public safety, but for the lives of the accused and crime victims, as well as their families and communities. And yet unconstrained judicial discretion can be dangerous, if judges are biased against a defendant or type of defendant, for example.
If risk assessments can make those decisions even somewhat easier, and do so in a rigorous, evidence-based manner, leading to fairer and more just outcomes, then they would be an unalloyed good. Proponents of the algorithms say that imposing some mathematical regularity will lead to greater transparency and accountability, and ultimately to an improvement on the current system.
But the reality of risk assessment algorithms is more complicated. Critics say bias can creep in at every stage, from development to implementation to application. Often that’s racial bias, but other characteristics such as age and ethnicity may also drive inequities.
These purportedly objective algorithms are developed by humans using imperfect data and are enmeshed in fraught political questions. They are also often used in situations beyond their stated intents. Risk assessments developed to measure whether someone will return to court are sometimes erroneously used to measure that person’s risk of reoffense, for example.
Despite the talismanic manner in which people deploy the word “algorithm,” nearly every aspect of such decision-making is ultimately dependent on human ingenuity (or lack thereof).
Since the age of the algorithmic risk assessment is underway, the question is not whether to use these tools, but how to do so in a way that maximizes fairness and minimizes harm. Even some observers who are wary of bias see promise in the use of risk assessments to inform treatment decisions and provide access to resources besides incarceration.
This explainer will help to make sense of some of the basic technical aspects of these tools, as well as the state of play with regard to the controversies that dominate the field.
What does it mean for a risk assessment tool to be “algorithmic”?
Risk assessments have been a part of the United States criminal legal system since at least the 1930s, but for decades they were clinical, meaning that they relied primarily on expert judgment. These experts may have been psychologists, social workers, probation officers, or other justice system actors, but the assessment hinged on the judgment of the person conducting it.
In contrast, actuarial risk assessments are based on statistical models and ostensibly do not rely on human judgment. Such models could be as straightforward as linear or logistic regression models like you may have learned in an introductory statistics class or could involve modern machine-learning techniques.
Algorithmic risk assessments, then, are just a kind of actuarial risk assessment. The term is typically used to refer to risk assessments where a computer performs a series of steps to provide the ultimate risk scoring.
Despite the talismanic manner in which people deploy the word “algorithm,” nearly every aspect of such decision-making is ultimately dependent on human ingenuity (or lack thereof). Algorithms are designed by humans, run on computers that humans built, trained on data that humans collect, and evaluated based on how well they reflect human priorities and values. Despite the utility of this approach, one theme that recurs in stories of algorithmic justice is how thoroughly human flaws pervade ostensibly objective mechanical processes.
How do risk assessment algorithms work?
Most risk assessment tools combine court and demographic records with some sort of questionnaire administered by a court official, such as a pretrial services officer in a bail context, or a prison social worker in a parole determination. Some tools, such as the Public Safety Assessment created by the Arnold Foundation (now known as Arnold Ventures), omit the questionnaire and use only the static data. The tools consider such things as criminal history, job status, level of education, and family information, giving each a numerical weight that makes the factor more or less important in the calculation of the final score.
The idea of weights in the model bears special emphasis, because in many cases that is the least transparent part of the algorithm. Although some creators of risk assessments share details of their models with the public, other models are developed by private companies that keep the inner workings of their algorithms secret. Even for some of the most locked-down, proprietary tools, the questionnaires that practitioners use to administer them are freely available, as are training manuals. The Arnold Foundation’s Public Safety Assessment uses these factors, which are generally representative of the field:

The process of setting the weights is known as training the model. In the machine learning context, this is known as supervised learning. A data set is fed into the model that contains a set of features, such as age, number of prior arrests, etc., and a set of known values for the outcome variable the model is supposed to predict.
For instance, if the model is supposed to predict likelihood of rearrest within two years (as it is in many risk assessment tools), the data used to train the model would have a big data set formatted much like a spreadsheet that associated values of the input variables with a value for the output variable (i.e., prediction). The input used to train a simple model using just the age and number of prior arrests used to predict rearrest might look like:

A real data set would be much bigger and have many more variables, but here the process of setting the weights for this simple model would just involve figuring out how important age is in conjunction with number of prior arrests in predicting the likelihood of a given outcome (rearrest within two years or not). Once the developer has established these weights (and ideally after a great deal of testing), the model could be applied to new data.
Algorithmic data, human priorities
Even the basic model described above, with two features and one output, introduces two of the most contentious issues with algorithmic risk assessments, namely the choice of inputs and the quality of the data. The choice of inputs reflects both what the tool developers aim to predict as well as the data they believe will make those predictions accurate. Similarly, the quality of the data that goes into the model reflects the priorities of the people who collect the data, i.e. what they choose to capture.
But there is often a mismatch between the outcome a risk assessment is meant to predict and the data used to train the predictive model, and this gap is largely due to the nature of the available data. Data about arrests and convictions are abundant, making it an attractive choice for tool developers. However, arrest data show more about the behavior of law enforcement and courts than about the individuals to whom the tools are applied.
A risk assessment that is used to inform a sentencing decision ought to predict whether the person being sentenced is going to commit a new crime at some point after the end of a period of supervision. And that decision should be based on whether people with similar profiles went on to commit crimes in the past. But while estimated data about such activities are available, those estimates are incomplete.
Many developers of risk assessment tools, such as the makers of COMPAS, use rearrest within two years as a definition of recidivism. As such, the models these developers build will be based on data containing just this statistic. But ideally, a tool designed to inform sentencing decisions ought to predict the likelihood that the person being sentenced will commit a new crime—not that that person will be arrested again. Many crimes occur for which no one is arrested, and of course people are routinely arrested without having committed a crime. The likelihood that someone will be arrested has at least as much to do with policing, which often disproportionately targets low-income communities of color, as much as it does with the behavior of the person getting sentenced.
This has been particularly problematic in Philadelphia. Bradley Bridge, an attorney from the Defender Association of Philadelphia, told The Marshall Project, “This is a compounding problem. … Once they’ve been arrested once, they are more likely to be arrested a second or a third time—not because they’ve necessarily done anything more than anyone else has, but because they’ve been arrested once or twice beforehand.” [Anna Maria Barry-Jester, Ben Casselman, and Dana Goldstein / The Marshall Project]
There are other problems embedded in the choice of stats. Risk assessments often use “failure to appear” rates to predict someone’s likelihood of returning to court, but most people who miss court aren’t intentionally dodging an appointment. They may be homeless, have child-care issues, or have simply forgotten. Using this data to predict whether someone will abscond yields misleading results. [The ‘Failure to Appear’ Fallacy, Ethan Corey and Puck Lo / The Appeal]
Similarly, an arrest on its own doesn’t necessarily mean a person reoffended.
Although arrests and convictions are an imperfect measure of actual criminal offending, they are used, as it were, on both sides of the equation. That is, nearly any model meant to predict recidivism will use arrests both as a predictor as well as the thing to be predicted.
As a recent paper from the AI Now Institute argues in the related context of predictive policing, building predictive models with data about policing risks laundering that data, turning it from a description of what has happened in the past to an algorithmic plan for what should happen in the future. As long as arrests are fed into risk assessments, the same danger applies. [Rashida Richardson, Jason Schultz, and Kate Crawford / SSRN]
Each context in which a risk assessment is used also raises unique data questions. Risk assessments are used at many stages of the criminal legal process, from bail to sentencing to parole determinations, and measuring risk means different things in each of these stages. For instance, the likelihood that a person will show up to court is irrelevant to a risk assessment tool used to inform parole decisions, but is of course essential in pretrial risk assessments.
By the same token, a sentencing risk assessment that predicts the likelihood of rearrest within two years would not be especially useful in the short-term horizon required for a pretrial tool. Despite this, risk assessment tools are often put to uses other than those for which they were designed. For instance, COMPAS was initially developed as software to help corrections departments manage cases, yet today it is commonly used in other contexts, such as in sentencing. [Danielle Kehl, Priscilla Guo and Samuel Kessler / Berkman Klein Center, Harvard Law School]
All of this is just to say that developers of risk assessment tools must consider the provenance of the data they use to train their models, lest they perpetuate the flaws of existing policing strategies while enshrining them in a veneer of mathematical inevitability. Similarly, consumers of risk assessment tools need to know about the choices the tool developers make, and to be aware both of the limitations in the data as well as the broader social context in which the data was created.
Are algorithmic risk assessments “biased”?
The question of bias in risk assessments captured headlines in 2016 when ProPublica published an article subtitled “There’s software used across the country to predict future criminals. And it’s biased against blacks.” The authors of the study thoroughly analyzed the application of COMPAS at sentencing in courts in Broward County, Florida. The analysis showed a racial disparity in false positive and false negative rates, meaning that people the algorithm determined to be “high-risk” but who were not rearrested within two years were more likely to be Black. Similarly, those who COMPAS labelled “low-risk” but did have a new arrest within two years, were more likely to be white.
So ProPublica’s fairness metric looked backward to see what the algorithm got right and wrong, and then compared the error rate across races. [Julia Angwin, Jeff Larson, Surya Mattu and Lauren Kirchner / ProPublica]
Northpointe (now called Equivant), the company that developed COMPAS, defended the tool, arguing that COMPAS was not biased because white people with high risk scores were about as likely as Black people with high risk scores to be rearrested. [Equivant]
But Northpointe’s notion of fairness misses something: Black and white people in Broward County (and elsewhere) are arrested at disparate rates, in part due to biased policing, so Black people are more likely to have arrests on their records. An algorithm that predicates the likelihood of reoffending based on arrest records perpetuates those same racial disparities.
“Crime rates are a manifestation of deeper forces,” University of Georgia law professor Sandra Mayson said. “Racial variance in crime rates, where it exists, manifests the enduring social and economic inequality that centuries of racial oppression have produced.” Codifying those historical inequities into algorithms may drive more incarceration, further compounding generations of discrimination. [Sandra Mayson / SSRN]
The question of whether Northpointe’s approach can still be “fair” is one for policymakers and, ultimately, for the communities whose values those policymakers represent. The potential public safety impact of false negatives will likely be prominent in such discussions, but false positives deserve as much or more attention.
In the pretrial context, a false positive may mean a person losing a job while being detained unnecessarily, or even make it more likely for that person to be ultimately convicted. For sentencing or parole determinations, false positives may lead to substantially longer periods of incarceration and more time away from their families and communities. The extent to which false positives destabilize the lives of the people affected—and the potential criminogenic impact of that destabilization—should not be discounted.
It’s also worth noting that bias may go undetected due to business interests of companies such as Northpointe, which refused to fully disclose its methods at the risk of losing a competitive advantage.
The problems of interpretation
One of the main arguments proponents of risk assessments put forward is that, even if there is some bias in the algorithms, that bias can be observed, studied, and corrected in ways that human bias cannot. In practice however, the interplay between algorithmic and human decision-making is complicated and sometimes leads to worse outcomes than either a human or computer deciding alone.
Kentucky’s experience with risk assessment shows some of the complexity involved when human and algorithmic judgment interact. In 2013, the commonwealth rolled out the Arnold Foundation’s Public Safety Assessment (PSA) with the express goal of lowering pretrial detention rates. Implementation of the tool came with a statutory presumption of release on nonmonetary conditions for low- and moderate-risk defendants. However, an analysis by George Mason University law professor Megan Stevenson of bail decisions in Kentucky in the risk assessment era demonstrated several troubling findings.
For one, racial disparities in pretrial release rates increased after the algorithm was adopted, largely due to the fact that judges in largely white, rural areas increased their release rates at a higher rate than judges in more diverse, nonrural areas. [Megan Stevenson / Minnesota Law Review]
Further, Stevenson’s analysis showed that early gains in decarceration due to the tool were short-lived. Immediately following PSA’s rollout, the pretrial detention rate in Kentucky plummeted, with 63 percent of “low-risk” defendants granted some form of non-monetary release. “Moderate-risk” individuals fared similarly. However, even after this dramatic decline, Kentucky still has a pretrial detention rate above the national average. [Megan Stevenson / Minnesota Law Review]
While better technology has the potential to make risk assessments fairer, that result is far from guaranteed.
California has also had a troubling history with pretrial risk assessments, also largely due to judges selective application of the tools’ recommendations. For instance, Human Rights Watch reported that in Santa Cruz County, “Judges agreed with 84 percent of the ‘detain’ recommendations, but just 47 percent of ‘release’ recommendations.” Similarly, Alameda County judges set bail on three-fourths of defendants labeled “low-risk.” [Human Rights Watch]
What accounts for these judges’ tendency to disproportionately override risk determinations that would have been favorable to defendants or the accused? One recent study suggests that poverty may interact with risk scores in the sentencing context to produce harsher sentences for the economically disadvantaged.
More than 300 judges participated in a controlled experiment in which they were given vignettes describing defendants differing only in socioeconomic status (relatively poor vs. relatively affluent) and whether risk assessment information was provided. The judges were asked whether they would sentence the person to probation or incarceration. The study found that judges who received risk assessment information were 15 percentage points less likely to incarcerate an affluent defendant than those who did not see a risk score. Conversely, presenting risk information about a relatively poor defendant was associated with a 15 percentage point increase in likelihood of incarceration. [Jennifer L. Skeem, et al. / SSRN]
The very way in which risk assessment results are presented to judges may affect how they use those reports in their bail or sentencing determinations. Most risk assessment tools, rather than presenting numerical risk scores, present describe subjects as being “low,” “medium,” or “high” risk.
For instance, the Pretrial Risk Assessment (PTRA) used in the federal court system characterizes subjects as having a risk level between 1 and 5. Intuitively, one might think that each of these risk levels corresponds to 20 percentage points on the risk scale, such that those at level 1 risk are in the range of 1 to 20 percent likely to violate conditions of pretrial release, and that someone at level 5 is nearly certain to violate, at between 80 and 100 percent.

In fact, fewer than 20 percent of those in the highest risk group according to the PTRA will violate conditions of release by being charged with a new offense. A judge relying on the intuitive interpretation of these scores will dramatically overestimate not just the actual risk such a person may pose, but even just the output of the tool itself. [Laurel Eckhouse, et al. / Criminal Justice and Behavior]
There is some evidence to suggest that taking human judgment out of the risk assessment process entirely could provide better outcomes. A computer simulation using a model trained on five years of New York City arrest data showed that replacing human judgment in bail decisions with a risk assessment tool could reduce pretrial incarceration by 40 percent without affecting crime rates. In some versions of the model, the researchers were able to weed out some racial bias by ensuring that the number of jailed defendants of a certain race could not be higher than the percentage of defendants of that race. [Jon Kleinberg, et al. / NBER]
Other research suggests that machine learning algorithms can outperform human judges in determining likelihood of an accused person failing to appear in court, and that even much simpler algorithms perform nearly as well as the more complex machine learning models on this task. [Jongbin Jung, et al. / SSRN]
Conversely, a recent study found that untrained laypeople recruited through Amazon’s Mechanical Turk performed comparably to COMPAS at predicting recidivism risk. However, critics claim that methodological flaws call these results into question. [Julia Dressel and Hany Farid / Science Advances]
None of this is meant to suggest that computers should have sole discretion in bail or sentencing decisions. Not only is it extremely unlikely that any jurisdiction would take humans out of the loop entirely (if such a scenario were even desirable), but, as one state supreme court found, doing so could even violate the constitutional right to due process.
Algorithms go to court: The Loomis case
In the 2016 case State v. Loomis, the Wisconsin Supreme Court became the highest court to squarely address constitutional questions around risk assessments.
Eric Loomis pleaded guilty to several charges stemming from a 2013 drive-by shooting. The trial court then conducted a presentence investigation that included a COMPAS risk assessment.
Loomis was determined to be “high risk” across all of the dimensions that COMPAS purports to measure. He received a split sentence where he would have to serve at least six years in prison with a substantial period of supervision thereafter. Loomis then filed a postconviction motion challenging his sentence on the grounds that the use of COMPAS violated his right to due process (among other challenges that are not related to risk assessments). This challenge ultimately made its way to the state’s high court. [State v. Loomis]
Loomis had several objections to how COMPAS was used in his case. For one thing, he claimed he was unable to effectively challenge his risk score because of the closed, proprietary nature of the tool, and that this violated his right to be sentenced based on accurate information. The court was not troubled by this use of a black-box model, finding that Loomis’s due-process rights were satisfied by his ability to challenge the accuracy of the facts that were fed into the model as well as to present evidence that the risk scores COMPAS generated were incorrect as they applied to him. The court found that the risk assessment was not itself determinative of Loomis’s punishment, and was just one piece of information among many. [State v. Loomis]
While the court ultimately declined to grant Loomis relief, it did take the opportunity to articulate a set of disclaimers that must accompany risk assessment reports going forward, each of which raises important considerations for courts using algorithms to punish:
First, the court mandated that proprietary risk assessment tools note when they do not disclose the risk factors they use or the weights assigned to them. So while the court did not find that the use of secret algorithms impinged on Loomis’s rights, it nonetheless determined that Wisconsin judges ought to keep the opaque nature of these tools in mind when using them at sentencing.
Second, the court required that risk assessment reports come with caveats when the tools used to create them have not been subject to validation studies that verify their models’ predictions against the local population. This problem is not unique to Wisconsin. Most states that use risk assessments do not have such studies; those that do have not made their results available.
The court required further that reports acknowledge concerns about racial bias of the kind discussed above. Courts will undoubtedly return to this issue with increasing frequency as the tools become ubiquitous.
Just as courts need to be confident that risk assessments are correct with respect to the residents of their communities, they must ensure that the tools remain accurate over time. The failure to do so could result in so-called zombie predictions that may artificially overstate risk due to outdated data. The court’s fourth admonition explains just this risk. [John Logan Koepke and David G. Robinson / Washington Law Review]
The court’s final label warns of the danger of applying risk assessment tools to different contexts than those for which they were designed, such as using a recidivism tool to predict risk of failure to appear.
Some critics have dismissed the Loomis court’s mandated disclaimer as “window dressing.” Indeed, the court could have gone further in actually addressing some of the shortcomings it observed rather than just requiring they be pointed out to judges going forward. However, if other courts that are considering risk assessments show the same willingness to engage with the subject as Wisconsin’s high court, there is room for optimism.
Conclusion
The use of algorithmic risk assessments has exploded in recent years, and despite the efforts of organizers who are opposed to the tools, this trend seems unlikely to abate any time soon. The technology is sure to become more sophisticated as well, which would lead to even less transparency as today’s relatively simple, interpretable models are supplanted by powerful but opaque deep learning methods.
While better technology has the potential to make risk assessments fairer, that result is far from guaranteed, and it is up to the people who design, implement, and employ these tools to ensure they do so in ways that reflect the values of their communities and safeguard the rights of those at society’s margins.